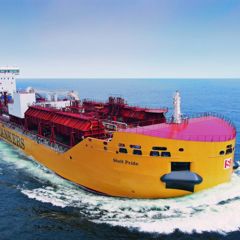
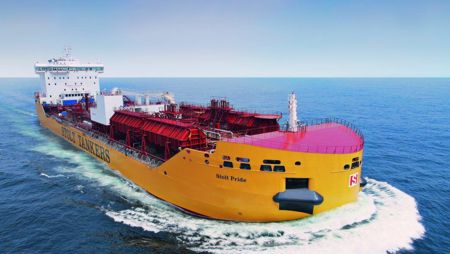
Why do companies hesitate when starting with smart maintenance?
Many organizations see the potential and are probably already planning to start smart maintenance. Yet, companies are hesitant to actually start. They often struggle with the same questions. Recognizable?
If you want to get past the ideas and proof-of-concept phase, determining the right use cases is essential: applications of smart maintenance that deliver immediate value.
Getting beyond the idea and proof-of-concept stages? Our answer: keep it small but think big.
Some tips:
Start with use cases that can be named concretely and approached pragmatically:
Don’t focus on the one, fatal mistake...
We see that many providers of smart maintenance technology focus on preventing that one fatal error. However, these errors are very rare in practice. Most companies for whom uptime is crucial have already optimized their production chain tremendously. Preventing that one failure, which may occur once every ten years, will therefore not quickly recoup your investment in predictive or smart maintenance.
Instead, it is better to look further into the indirect, perhaps ‘smaller’ effects, which do provide a high return on investment. Compare this to, say, the tire pressure of a car. Instead of doing everything possible to prevent a blowout that might happen once in a lifetime, it is better to ensure that you always drive with optimal tire pressure. This is safer and you will also save a lot of fuel, and therefore money.
“We suspected that it would be possible to make predictions about possible failures in the engines of our tankers. Our own team was unable to substantiate this expectation with a working algorithm. HSO’s approach did lead to a concrete, predictive model.”